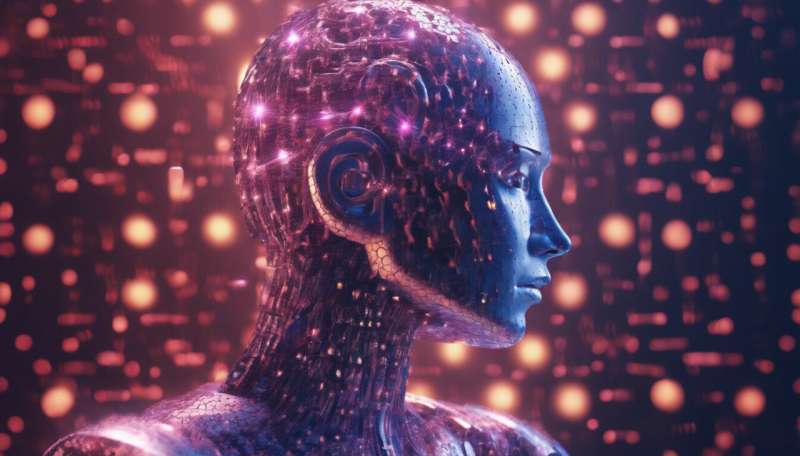
A public passion staff filed a U.S. federal criticism in opposition to synthetic intelligence hiring software, HireVue, in 2019 for misleading hiring practices. The instrument, which has been followed by means of masses of businesses, preferred sure facial expressions, talking types and tones of voice, disproportionately disadvantaging minority applicants.
The Digital Privateness Data Middle argued HireVue’s effects have been “biased, unprovable and no longer replicable.” Despite the fact that the corporate has since stopped the use of facial popularity, issues stay about biases in different biometric knowledge, equivalent to speech patterns.
In a similar way, Amazon stopped the use of its AI recruitment software, as reported in 2018, after finding it was once biased in opposition to girls. The set of rules, skilled on male-dominated resumes submitted over 10 years, preferred male applicants by means of downgrading programs that incorporated the phrase “girls’s” and penalizing graduates of ladies’s schools. Engineers attempted to deal with those biases, however may no longer ensure neutrality, resulting in the venture’s cancellation.
Those examples spotlight a rising worry in recruitment and choice: whilst some firms are the use of AI to take away human bias from hiring, it could actually incessantly make stronger and magnify current inequalities. Given the fast integration of AI into human useful resource control throughout many organizations, you need to lift consciousness concerning the complicated moral demanding situations it gifts.
Tactics AI can create bias
As firms increasingly more depend on algorithms to make vital hiring selections, it is a very powerful to pay attention to the next techniques AI can create bias in hiring:
1. Bias in coaching knowledge. AI techniques depend on huge datasets—known as coaching knowledge—to be informed patterns and make selections, however their accuracy and equity are most effective as excellent as the knowledge they’re skilled on. If this information comprises ancient hiring biases that choose explicit demographics, the AI will undertake and reproduce those self same biases. Amazon’s AI software, as an example, was once skilled on resumes from a male-dominated business, which ended in gender bias.
2. Wrong knowledge sampling. Wrong knowledge sampling happens when the dataset used to coach an set of rules isn’t consultant of the wider inhabitants it is intended to serve. Within the context of hiring, it will occur if coaching knowledge over-represents sure teams —normally white males—whilst under-representing marginalized applicants.
Consequently, the AI might learn how to choose the traits and studies of the over-represented staff whilst penalizing or overlooking the ones from underrepresented teams. As an example, facial research applied sciences have proven to have upper error charges for racialized folks, specifically racialized girls, as a result of they’re underrepresented within the knowledge used to coach those techniques.
3. Bias in function variety. When designing AI techniques, builders select sure options, attributes or traits to be prioritized or weighed extra closely when the AI is making selections. However those decided on options can result in unfair, biased results and perpetuate pre-existing inequalities.
As an example, AI may disproportionately price graduates from prestigious universities, that have traditionally been attended by means of other people from privileged backgrounds. Or, it would prioritize paintings studies which can be extra not unusual amongst sure demographics.
This downside is compounded when the options decided on are proxies for safe traits, equivalent to zip code, which will also be strongly associated with race and socioeconomic standing because of ancient housing segregation.
4. Loss of transparency. Many AI techniques serve as as “black bins,” that means their decision-making processes are opaque. This loss of transparency makes it tricky for organizations to spot the place bias may exist and the way it impacts hiring selections.
With out perception into how an AI software makes selections, it is tricky to right kind biased results or be sure that equity. Each Amazon and HireVue confronted this factor; customers and builders struggled to know the way the techniques assessed applicants and why sure teams have been excluded.
5. Loss of human oversight. Whilst AI performs a very powerful function in lots of decision-making processes, it must increase, quite than exchange, human judgment. Over-reliance on AI with out ok human oversight can result in unchecked biases. This downside is exacerbated when hiring execs agree with AI greater than their very own judgment, believing within the era’s infallibility.
Overcoming algorithmic bias in hiring
To mitigate those problems, firms will have to undertake methods that prioritize inclusivity and transparency in AI-driven hiring processes. Underneath are some key answers for overcoming AI bias:
1. Diversify coaching knowledge. Probably the most efficient techniques to fight AI bias is to make sure coaching knowledge is inclusive, numerous and consultant of a variety of applicants. This implies together with knowledge from numerous racial, ethnic, gender, socioeconomic and academic backgrounds.
2. Habits common bias audits. Common and thorough audits of AI techniques must be performed to spot patterns of bias and discrimination. This comprises inspecting the set of rules’s outputs, decision-making processes and its affect on other demographic teams.
3. Put into effect fairness-aware algorithms. Use AI instrument that comprises equity constraints and is designed to believe and mitigate bias by means of balancing results for underrepresented teams. This may come with integrating equity metrics equivalent to equivalent alternative, enhancing coaching knowledge to turn much less bias and adjusting type predictions in response to equity standards to extend fairness.
4. Build up transparency. Search AI answers that supply perception into their algorithms and decision-making processes to assist you determine and cope with attainable biases. Moreover, you’ll want to expose any use of AI within the hiring procedure to applicants to care for transparency along with your task candidates and different stakeholders.
5. Care for human oversight. To care for regulate over hiring algorithms, managers and leaders will have to actively assessment AI-driven selections, particularly when making ultimate hiring alternatives. Rising analysis highlights the vital function of human oversight in safeguarding in opposition to the dangers posed by means of AI programs. Then again, for this oversight to be efficient and significant, leaders will have to make sure that moral concerns are a part of the hiring procedure and advertise the accountable, inclusive and moral use of AI.
Bias in hiring algorithms raises severe moral issues and calls for better consideration to the conscious, accountable and inclusive use of AI. Working out and addressing the moral concerns and biases of AI-driven hiring is very important to making sure fairer hiring results and combating era from reinforcing systemic bias.
Equipped by means of
The Dialog
This newsletter is republished from The Dialog beneath a Ingenious Commons license. Learn the unique article.
Quotation:
When AI performs favorites: How algorithmic bias shapes the hiring procedure (2024, October 14)
retrieved 14 October 2024
from https://phys.org/information/2024-10-ai-plays-favorites-algorithmic-bias.html
This report is matter to copyright. Excluding any truthful dealing for the aim of personal learn about or analysis, no
section is also reproduced with out the written permission. The content material is equipped for info functions most effective.