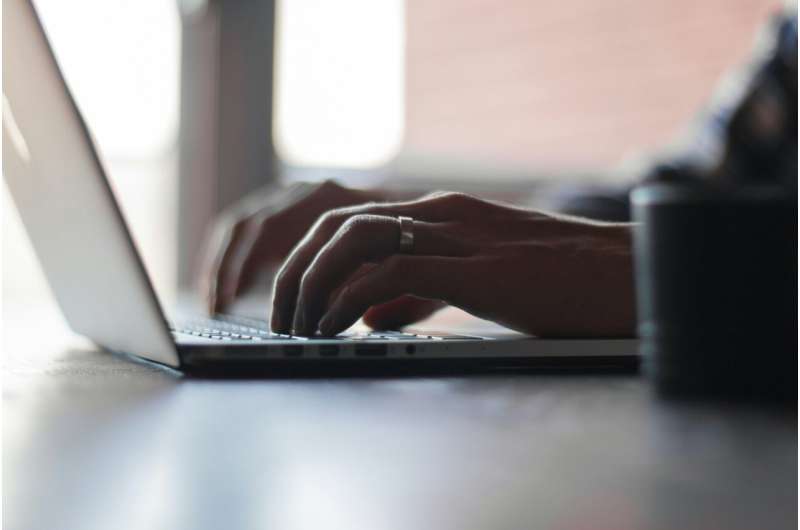
Wading through the staggering amount of social media content being produced every second to find the nastiest bits is no task for humans alone.
Even with the newest deep-learning tools at their disposal, the employees who identify and review problematic posts can be overwhelmed and often traumatized by what they encounter every day. Gig-working annotators who analyze and label data to help improve machine learning can be paid pennies per unit worked.
In a Concordia-led paper published in IEEE Technology and Society Magazine, researchers argue that supporting these human workers is essential and requires a constant re-evaluation of the techniques and tools they use to identify toxic content.
The authors examine social, policy, and technical approaches to automatic toxicity detection and consider their shortcomings while also proposing potential solutions.
“We want to know how well current moderating techniques, which involve both machine learning and human annotators of toxic language, are working,” says Ketra Schmitt, one of the paper’s co-authors and an associate professor with the Centre for Engineering in Society at the Gina Cody School of Engineering and Computer Science.
She believes that human contributions will remain essential to moderation. While existing automated toxicity detection methods can and will improve, none is without error. Human decision-makers are essential to review decisions.
“Moderation efforts would be futile without machine learning because the volume is so enormous. But lost in the hype around artificial intelligence (AI) is the basic fact that machine learning requires a human annotator to work. We cannot remove either humans or the AI.”
Arezo Bodaghi is a research assistant at the Concordia Institute for Information Systems Engineering and the paper’s lead author. “We cannot simply rely on the current evaluation matrix found in machine and deep learning to identify toxic content,” Bodaghi adds. “We need them to be more accurate and multilingual as well.
“We also need them to be very fast, but they can lose accuracy when machine learning techniques are fast. There is a trade-off to be made.”
Broader input from diverse groups will help machine-learning tools become as inclusive and bias-free as possible. This includes recruiting workers who are non-English speakers and come from underrepresented groups such as LGBTQ2S+ and racialized communities. Their contributions can help improve the large language models and data sets used by machine-learning tools.
Keeping the online world social
The researchers offer several concrete recommendations companies can take to improve toxicity detection.
First and foremost is improving the working conditions for annotators. Many companies pay them by the unit of work rather than by the hour. Furthermore, these tasks can be easily offshored to workers demanding lower wages than their North American or European counterparts, so companies can wind up paying their employees less than a dollar an hour.
And little in the way of mental health treatment is offered even though these employees are front-line bulwarks against some of the most horrifying online content.
Companies can also deliberately build online platform cultures that prioritize kindness, care, and mutual respect as opposed to others such as Gab, 4chan, 8chan, and Truth Social, which celebrate toxicity.
Improving algorithmic approaches would help large language models reduce the number of errors made around misidentification and differentiating context and language.
Finally, corporate culture at the platform level has an impact at the user level.
When ownership deprioritizes or even eliminates user trust and safety teams, for instance, the effects can be felt company-wide and risk damaging morale and user experience.
“Recent events in the industry show why it is so important to have human workers who are respected, supported, paid decently, and have some safety to make their own judgments,” Schmitt concludes.
More information:
Arezo Bodaghi et al, Technological Solutions to Online Toxicity: Potential and Pitfalls, IEEE Technology and Society Magazine (2024). DOI: 10.1109/MTS.2023.3340235
Citation:
Online toxicity can only be countered by humans and machines working together, say researchers (2024, February 28)
retrieved 24 June 2024
from https://techxplore.com/news/2024-02-online-toxicity-countered-humans-machines.html
This document is subject to copyright. Apart from any fair dealing for the purpose of private study or research, no
part may be reproduced without the written permission. The content is provided for information purposes only.