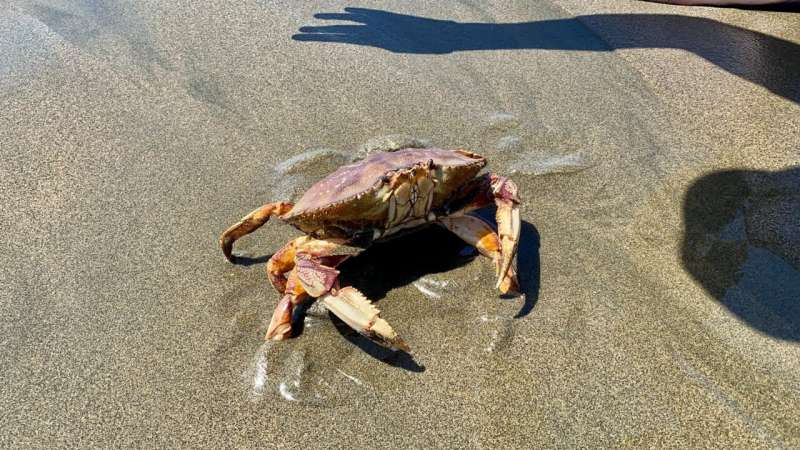
For the sixth consecutive year, California officials are delaying the Bay Area’s commercial Dungeness crab season because of the “high abundance” of migrating humpback whales already getting ensnared by old crab-pot fishing lines and other gear—while conservation groups are again expressing frustration with what they say is a too-slow transition to safer fishing methods.
The upcoming season normally would start Nov. 15 in the waters from the Sonoma/Mendocino County line south to the Mexican border.
The decision came down on Friday afternoon from Charlton H. Bonham, director of the California Department of Fish and Wildlife, and was made in consultation with representatives of the fishing industry, environmental organizations and scientists.
According to the nonprofit conservation group Oceana, a participant in those discussions, during the period from May to Oct. 21 of this year, four humpback whales were confirmed entangled in California commercial Dungeness crab fishing gear. Ten others were observed entangled in “unknown fishing gear that may be Dungeness crab gear.”
Other entanglements were sighted the past few days, said marine scientist Caitlynn Birch, Oceana’s campaign manager.
Both she and representatives from the Center for Biological Diversity spoke out in favor of the use of rope-less or pop-up gear to give whales a safe migration south.
“I’m glad state officials are taking precautions to avoid entangling whales in the area, but with viable pop-up gear available, these season delays are getting increasingly archaic,” said Ben Grundy, oceans campaigner at the Center for Biological Diversity, in a statement.
He noted that if the state had authorized the use of pop-up gear—which he said has performed well in tests—”crab fishers could be prepping to put their traps in the water right now.”
Although another assessment will take place on or around Nov. 22, that date will make it impossible for crab to make an appearance on Thanksgiving dinner tables on Nov. 28. That assessment could, however, lead to an opening date in early December.
The recreational Dungeness season will be allowed to start as scheduled on Nov. 2—but with restrictions. Crabbers may not use trap gear, according to the state order; only hoop nets and crab snares will be allowed until further assessment.
Since 2015, there have been delays in all but one commercial Dungeness season in the Bay Area. A toxin, domoic acid, that could sicken anyone who eats the tainted crab destroyed Northern California’s 2015–2016 commercial season and created delays in other years.
In 2018, the commercial season began without a hitch although recreational crabbers had to postpone their fishing.
In 2019 and 2020, the fishing line danger to whales resulted in a crabbing delay of several weeks. The 2020 crabbing season was officially set to begin Dec. 23, but price negotiations between crab fleets and seafood processors delayed the start until early January 2021.
With delays to protect whales, the truncated 2021–22 season ran from Dec. 29 to April 8, and the 2022–23 season from Dec. 31 to April 15. The 2023–24 season didn’t begin until Jan. 18, 2024, and closed early, on April 8.
2024 MediaNews Group, Inc. Distributed by Tribune Content Agency, LLC.
Citation:
California’s 2024 Dungeness crab season postponed to protect whales (2024, October 28)
retrieved 28 October 2024
from https://phys.org/news/2024-10-california-dungeness-crab-season-postponed.html
This document is subject to copyright. Apart from any fair dealing for the purpose of private study or research, no
part may be reproduced without the written permission. The content is provided for information purposes only.